Detection and classification of rainfall in South America using satellite images and machine learning techniques
DOI:
https://doi.org/10.4279/pip.150006Keywords:
Atmospheric Physics, Quantitative Precipitation Estimation, Convolutional Neural Networks, XGBoost, Machine Learning, Remote Sensing, Rainfall, Satellite InformationAbstract
The study of precipitation is one of the most intriguing areas in atmospheric sciences, with significant implications for our daily lives and climate change projections. This paper explores the estimation of rainfall trends in South American regions using convolutional neural networks (CNNs). The study focuses on the application of Cloud-Net, a CNN-based model with a format similar to an autoencoder, to obtain qualitative estimates of precipitation patterns. The employed loss functions, Categorical Cross Entropy and Categorical Focal Loss, address the challenges of classifying minority categories in unbalanced data. Regional analysis was conducted, identifying days with high rainfall intensity and the predominant intensities in 25 regions. The CNN model’s performance was compared with the XGBoost algorithm, showing excellent results for extreme rainfall categories and challenging intermediate categories. Furthermore, a comparison was made with Quantitative Precipitation Estimation (QPE) data and ground measurements from rain gauges. While the CNN model provided a valuable qualitative estimate of precipitation trends, achieving precise quantitative estimation would require an extensive data set of in-situ measurements. Overall, this research demonstrates the potential of CNNs for estimating rainfall trends and understanding precipitation patterns in South American regions. The findings offer valuable insights for further applications in meteorology and environmental studies.
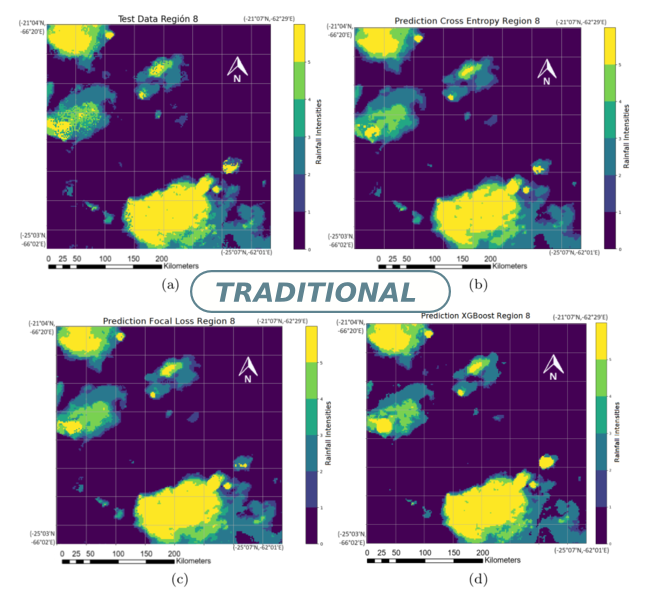
Published
How to Cite
Issue
Section
License
Copyright (c) 2023 Federico Andelsman, Sergio Masuelli, Francisco Tamarit

This work is licensed under a Creative Commons Attribution 4.0 International License.
Authors agree to the PIP Copyleft Notice